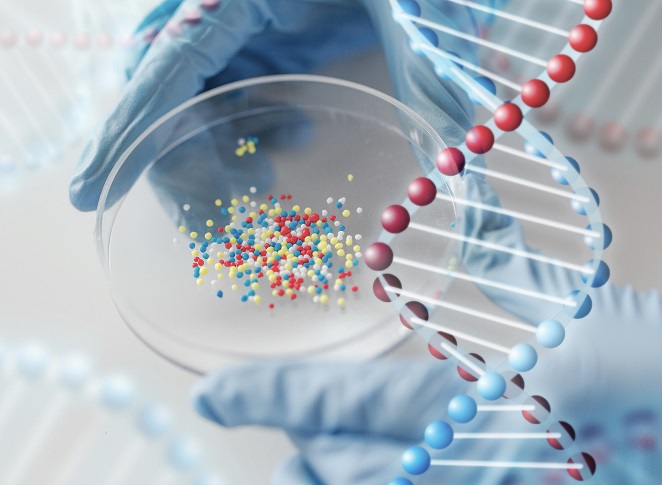
A biomarker is a response to a certain intervention or disease stage and is an indicator of a biological state. It can indicate molecular changes related to diseases; therefore, it can be used for disease diagnosis and the definition of therapeutic targets. Advances in genomic profiling technology and targeted molecular therapies have proved the importance of cancer biomarker discovery. Biomarkers are used to predict the type, stage and development of cancer, as well as to discover drugs by predicting the efficacy of various drugs against the tumors.
Data from various omics techniques are useful starting points for a biomarker discovery study. As huge amounts of omics data are available and since biomarkers play important roles in cancer development, using machine learning for the discovery of cancer biomarkers is important. Various feature selection algorithms have been applied to gene expression data to discover genes that are influential in cancer diagnosis and prognosis. Algorithms have also been developed to integrate multi-omics data in problems such as survival and treatment response prediction.
In this project, we investigate the development of ML algorithms which aim to discover different cancer biomarkers from genomics data. In addition, we study cancer type and sub-type classification and prediction of cancer-related outcomes from genomics data.